Profiling the Torrust BitTorrent UDP Tracker
Dive into the advanced profiling techniques driving the Torrust BitTorrent Tracker's performance. This post uncovers the tools and methods we leverage to ensure the tracker's efficiency, scalability, and our journey towards optimizing peer-to-peer file sharing.
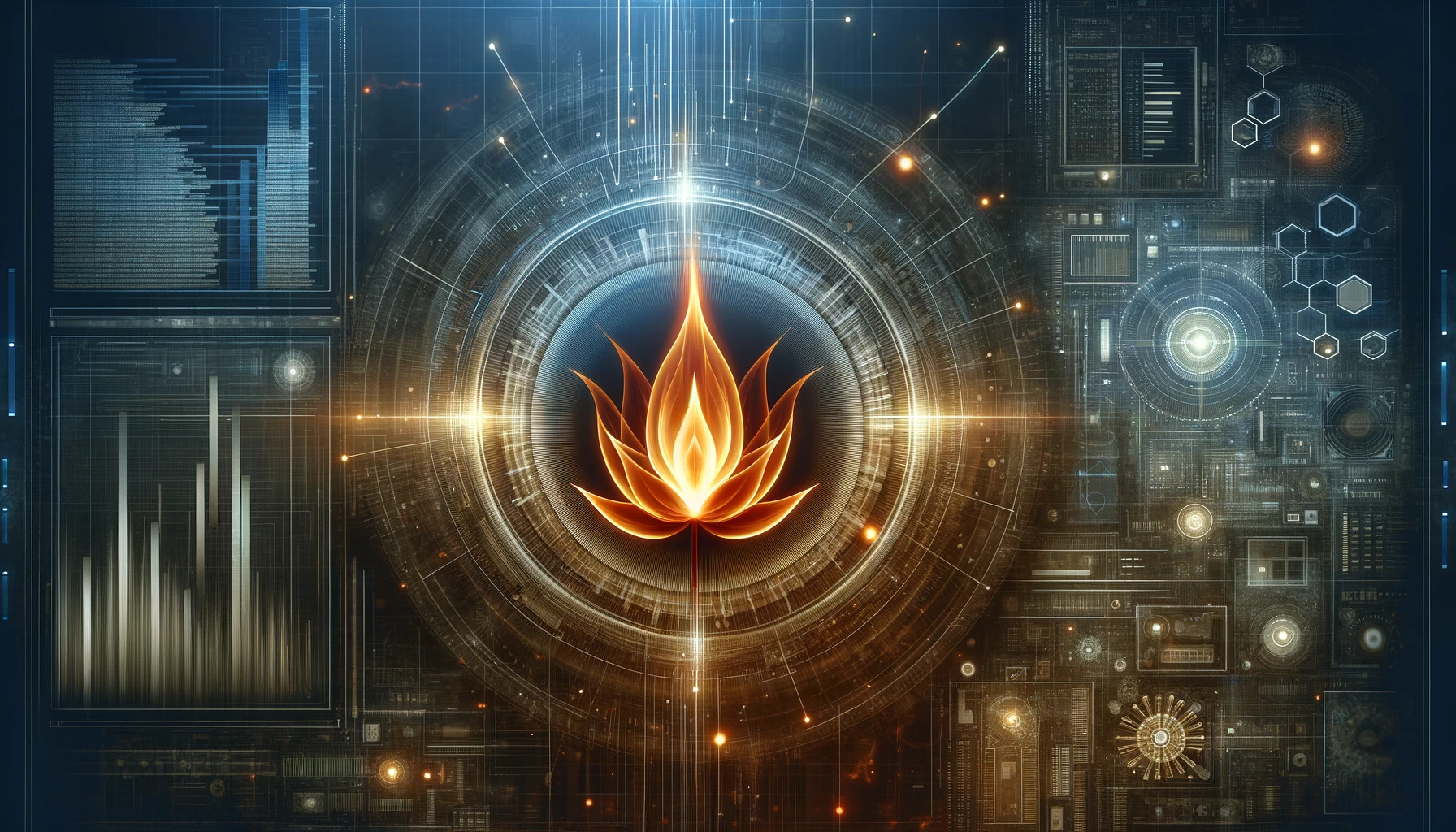
Introduction
In our quest to elevate the Torrust BitTorrent Tracker's performance, we've embraced a suite of sophisticated benchmarking and profiling tools. This article builds on our previous discussion, "Benchmarking the Torrust BitTorrent Tracker" and delves into the metrics that guide our optimization efforts. Join us as we explore the intricacies of profiling in the dynamic landscape of BitTorrent technology.
In the previous article we introduced to the community the benchmarking tools we are using at the moment to know whether the Tracker performs at the same level as other applications on the market.
With those benchmarking tools we ensure that the Tracker has a good performance and we don't have regressions. If you are curious about how we do benchmarking you can read the "Benchmarking the Torrust BitTorrent Tracker" article.
In this article we will explain how we are collecting metrics to know which parts of the code we should improve.
Understanding The Tracker
Before you continue reading this article you should know a little bit about the tracker internal structure. You can see an overview on the "Benchmarking the Torrust BitTorrent Tracker" article.
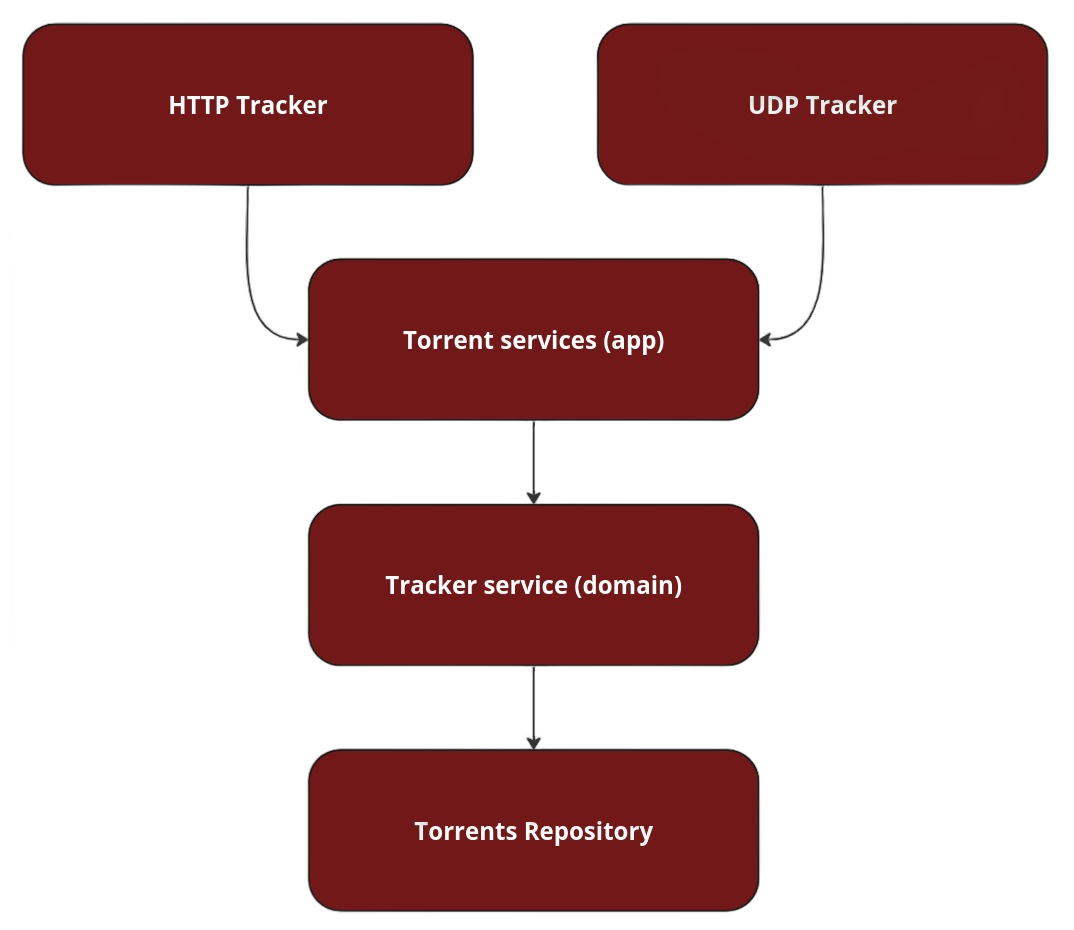
Current Challenges
Balancing feature-rich functionality with performance is our central challenge. The Torrents Repository, a critical component, has been our focus, serving as the primary bottleneck for scaling request handling capabilities.
Regardless which protocol clients use to connect to the tracker, eventually all requests reach the internal "Tracker" domain service. This tracker contains a repository with the list of all torrents. Each entry on the list contains two pieces of information:
- The statistics about that torrent: number of seeders, leechers and peers that have completed downloading.
- The peer list: a list of all the clients announcing the same torrent (swarm).
{
"info_hash": "090c6d4fb3a03191c4ef1fda6236ef0efb2d5c10",
"seeders": 1,
"completed": 1,
"leechers": 0,
"peers": [
{
"peer_id": {
"id": "0x2d71423030303030303030303030303030303031",
"client": null
},
"peer_addr": "0.0.0.0:17548",
"updated": 1709916034742,
"updated_milliseconds_ago": 1709916034742,
"uploaded": 0,
"downloaded": 0,
"left": 0,
"event": "Completed"
}
]
}
The reason why we have been focusing on that part is because we think that's the main bottleneck if we want to increase the number of requests the tracker can handle per second.
The announce
request is the most important request a tracker needs to handle.
Peers get the list of other peers from the tracker by making announce requests. The purpose
of that request is:
- To include the peer making the request in the list of peers which are seeding or downloading the torrent.
- Return the list of other peers so that the client can start asking for torrent pieces to the other peers.
Every request is a write/read request. The system is intensive in writes. All requests eventually try to acquire a write lock to include themselves in the peer list. That's why we think that's the main bottleneck in our implementation, at the moment. For that reason we have been trying different implementations of the torrents repository:
pub type TorrentsRwLockStd = RwLockStd<EntrySingle>;
pub type TorrentsRwLockStdMutexStd = RwLockStd<EntryMutexStd>;
pub type TorrentsRwLockStdMutexTokio = RwLockStd<EntryMutexTokio>;
pub type TorrentsRwLockTokio = RwLockTokio<EntrySingle>;
pub type TorrentsRwLockTokioMutexStd = RwLockTokio<EntryMutexStd>;
pub type TorrentsRwLockTokioMutexTokio = RwLockTokio<EntryMutexTokio>;
pub type TorrentsSkipMapMutexStd = CrossbeamSkipList<EntryMutexStd>; // Default
pub type TorrentsSkipMapMutexParkingLot = CrossbeamSkipList<EntryMutexParkingLot>;
pub type TorrentsSkipMapRwLockParkingLot = CrossbeamSkipList<EntryRwLockParkingLot>;
pub type TorrentsDashMapMutexStd = XacrimonDashMap<EntryMutexStd>;
The default implementation used in production is TorrentsSkipMapMutexStd
.
BTreeMap
or SkipMap
. The reason we use those types is because
the tracker API has an endpoint where you can get the ordered list of all torrents in the
tracker repository.Internal Repository Benchmarking
As we explained in a previous article ("Benchmarking the Torrust BitTorrent Tracker") you can run the benchmark for those different repository implementations with the following command:
cargo bench -p torrust-tracker-torrent-repository
The output at the time of writing this post is similar to:
Running benches/repository_benchmark.rs (target/release/deps/repository_benchmark-a9b0013c8d09c3c3)
add_one_torrent/RwLockStd
time: [63.057 ns 63.242 ns 63.506 ns]
Found 12 outliers among 100 measurements (12.00%)
2 (2.00%) low severe
2 (2.00%) low mild
2 (2.00%) high mild
6 (6.00%) high severe
add_one_torrent/RwLockStdMutexStd
time: [62.505 ns 63.077 ns 63.817 ns]
Found 11 outliers among 100 measurements (11.00%)
4 (4.00%) high mild
7 (7.00%) high severe
Benchmarking add_one_torrent/RwLockStdMutexTokio: Collecting 100 samples in estimated 1.0004 s (10M iterations)
add_one_torrent/RwLockStdMutexTokio
time: [98.440 ns 98.551 ns 98.660 ns]
Found 4 outliers among 100 measurements (4.00%)
3 (3.00%) low mild
1 (1.00%) high severe
add_one_torrent/RwLockTokio
time: [107.84 ns 108.18 ns 108.54 ns]
Found 3 outliers among 100 measurements (3.00%)
2 (2.00%) low mild
1 (1.00%) high mild
Benchmarking add_one_torrent/RwLockTokioMutexStd: Collecting 100 samples in estimated 1.0001 s (8.7M iterations)
add_one_torrent/RwLockTokioMutexStd
time: [116.34 ns 116.48 ns 116.63 ns]
Found 2 outliers among 100 measurements (2.00%)
1 (1.00%) high mild
1 (1.00%) high severe
Benchmarking add_one_torrent/RwLockTokioMutexTokio: Collecting 100 samples in estimated 1.0005 s (6.9M iterations)
add_one_torrent/RwLockTokioMutexTokio
time: [143.39 ns 143.51 ns 143.63 ns]
Profiling With Valgrind
"Valgrind is an instrumentation framework for building dynamic analysis tools".
In fact it's a suite of tools. The tool we are going to use is callgrind
which collects data that can be later visualized with kcachegrind
.
Valgrind, coupled with Kcachegrind, offers a powerful profiling solution for in-depth analysis of BitTorrent tracker performance. By simulating cache behavior and collecting call graphs, developers gain valuable insights into code execution dynamics and potential optimizations.
In order to profile the UDP tracker you need to:
- Build and run the tracker for profiling.
- Make requests to the tracker while it's running.
Build and run the binary for profiling with: